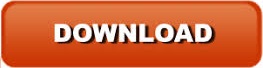
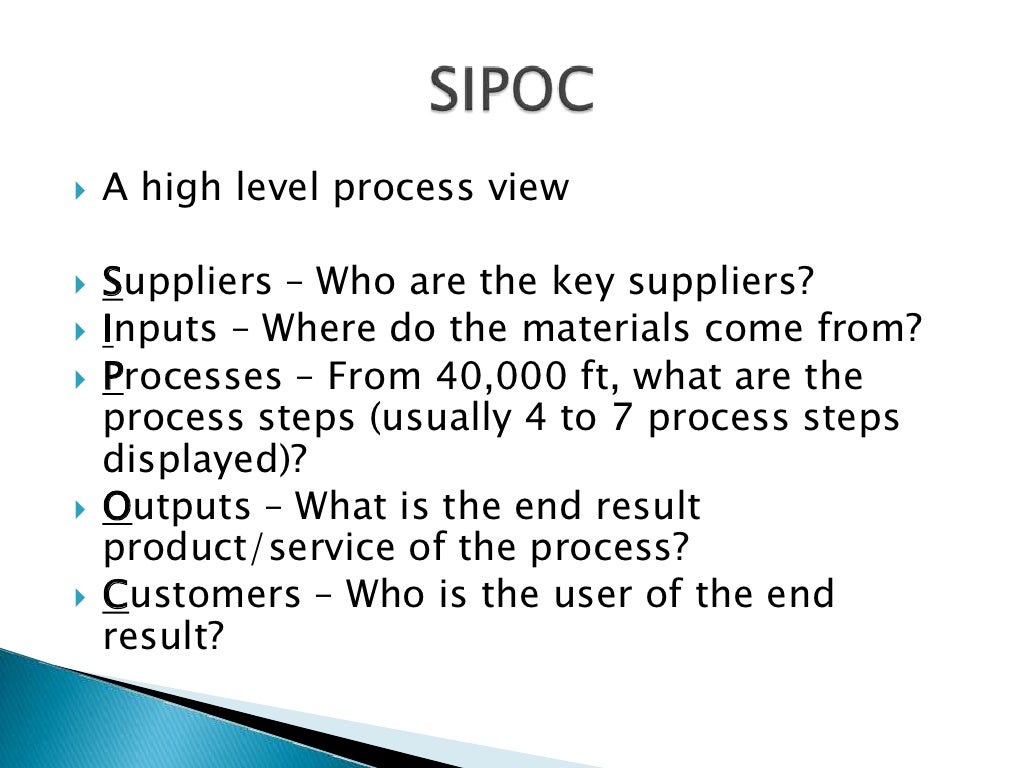
The standard deviation becomes an essential tool when testing the likelihood of a hypothesis. Around 0.1% of the population is 4 standard deviations from the mean, the geniuses. About 2.1 percent of the population is 3 standard deviations from the mean (3-sigma) - these are brilliant people. Data suggests 68 percent of the population are in what is called one standard deviation from the mean (one-sigma) and 27.2 percent of the population are two standard deviations from the mean, being either bright or rather intellectually challenged depending on the side of the bell curve they are on.
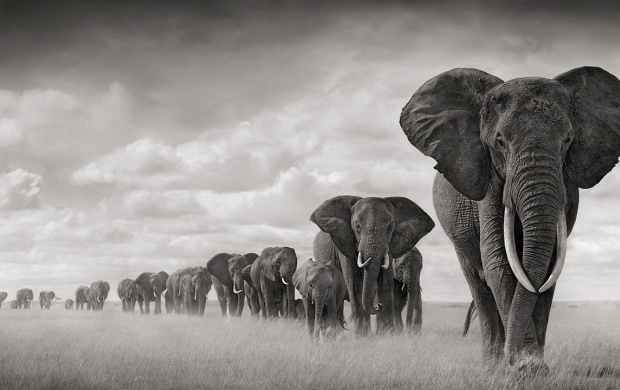
Two-sigma includes 95 percent and three-sigma includes 99.7 percent. Higher sigma values mean that the discovery is less and less likely to be accidentally a mistake or ‘random chance’. One standard deviation or one-sigma, plotted either above or below the average value, includes 68 percent of all data points. This is what you’d call a normal distribution, while the deviation is how far a given point is from the average. If you plot all of these coin-toss tests on a graph, you should typically see a bell-shaped curve with the highest point of the curve in the middle, tapering off on both sides. Sometimes you’ll get something like 45 vs 55 and in a couple of extreme cases 20 vs 80. If you repeat this 100-coin-toss test another 100 times, you’ll get even more interesting results. Rather, you’ll likely get something like 49 vs 51. If you flip a coin 100 times, though, chances are you won’t get 50 instances of heads and 50 of tails. The coin only has two sides, heads or tails, so the probability of getting one side of the other following a toss is 50 percent. To understand how scientists use the standard deviation in their work, it helps to consider a familiar statistical example: the coin toss. A low standard deviation, however, revolves more tightly around the mean.

Samples with a high standard deviation are considered to be more spread out, meaning it has more variability and the results are more interpretable. Typically denoted by the lowercase Greek letter sigma (σ), this term describes how much variability there is in a given set of data, around a mean, or average, and can be thought of as how “wide” the distribution of points or values is. When referring to statistical significance, the unit of measurement of choice is the standard deviation. But in order for a statistical result to be significant for everyone involved, you also need a standard to measure things. Namely, a result will be meaningful if it’s statistically significant. A skeptical outlook will always do you good but if this is the case how can scientists tell if their results are significant in the first place? Well, instead of relying on gut feeling, any researcher that’s worth his salt will let the data speak for itself. When doing science, you can never afford certainties.
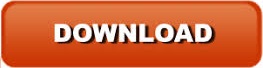